Monitoring, Learning and Evaluation (MLE) approaches for development interventions have been constantly evolving over time. It started as a country-level focus in the 1960s and expanded to individual program-level MLE approaches, methods and standards in the 1990s and newer and innovative approaches have been developed since then. Meanwhile, program design has evolved from being focused on a single intervention with a linear causal path to programs with multiple interventions, multiple implementing partners and multiple levels of implementation. For assessing these programs, complexity-aware MLE approaches have been developed and widely used in recent years. This blog highlights the MLE approach for 3ie’s Swashakt program and what we are learning from being more conscious of context and complexity.
Unpacking Complexity
3ie’s Swashakt program is supporting interventions to identify what works to enhance viability, scalability and returns of women’s collective enterprises and promote women’s economic empowerment. LEAD at Krea University is the grants management partner for the program, and has been working closely with the program team to develop its MLE framework. The program was launched in 2020 and has reached more than 6,900 women across 480 villages in 10 Indian states.
As a portfolio of nine projects working with a variety of collectives in different sectors, some in partnership with government agencies, Swashakt is a complex program (Figure 1). Each project has its own set of interventions, beneficiary type and local socio-cultural context. The infographic below presents the breadth of this complex multi-actor and multi-sector implementation and evidence generation program.
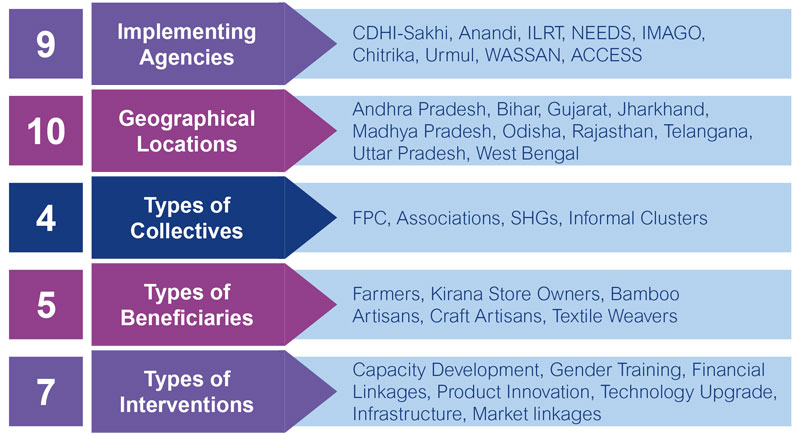
Our Approach
Keeping in mind the complexity of the Swashakt program, we adopted a participatory and inclusive MLE approach in which the project implementers have voice. At inception stage, we came up with the following four guiding principles for our approach (Figure 2):
- Inclusive – All stakeholders including the implementing agencies should be active partners in the MLE system, including in the design phase.
- Flexible – The MLE methods and indicators will not be rigid. There will be scope to modify them based on field learning.
- Learning-oriented – The learning component of MLE will not be a stand-alone one for sharing findings at the end of the program. Learning will be shared for course correction and adaptation through regular peer learning meets and review mechanisms.
- Gender-responsive – All indicators of monitoring and evaluation should be gender responsive.
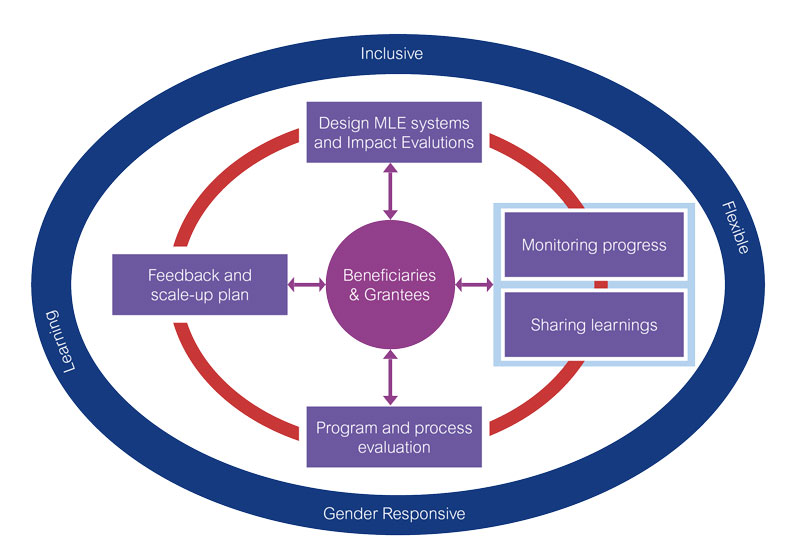
Complexity-aware Monitoring
Our guiding principles meant that while designing the monitoring framework, we collect data that provides insight into the overall progress of the project but requires minimum resources from the grantees. We co-developed the monitoring indicators, definitions and frequency of reporting through a workshop, with the grantees playing the lead role and us supporting them. We were cognizant of the following factors while developing the monitoring framework.
- Multiple partners, components and objectives
- The need to monitor both program-level and project-level indicators
- Measuring gender-sensitive indicators both at individual and enterprise level
- Monitoring blind spots of different projects
- The varying pace of change for different projects
- The capacity of individual grantees to implement monitoring systems
The final shortlist of monitoring indicators was based on the level of importance and feasibility of grantees assigned to each indicator. Once the implementation started, we held monthly check-in calls with each grantee after they shared the monitoring data to assess progress, validate data and course correct where required.
Learnings From Our Complexity-Aware Monitoring Approach
Our journey of implementing a complexity-aware, participatory MLE approach has not been a smooth ride. We faced challenges in adapting to the COVID-19 pandemic, and retraining the grantees on specific aspects of monitoring and collecting data on complex indicators. We have been able to overcome some of these challenges through our approach and are excited about the learnings we are generating, which will potentially help implementers to create impact and the broader research community to understand what works for women collectives. More than 18 months into the program, here are some of our key learnings:
1. Participatory Processes Must Be Inclusive
We found that involving all stakeholders, including ground-level project staff, in visioning and planning for the program increased their ownership and motivation, as well as reducing the risk that monitoring stops with attrition. By making the grantees and their teams take the lead, we made sure that the monitoring data is relevant for their own project review, planning and course correction.
2. Building Capacity Without Overburdening Fledgling Systems
The key to reducing the reporting stress of the grantees is to understand the minimum set of data points necessary for program review. Making sure that there are no additional tedious data collection efforts for grantees helps in getting timely and accurate data.
3. Importance of Feedback Loops
With implementation uncertainties caused due to COVID-19 pandemic, regular reviews of monitoring data helped us course correct where required. Implementing agencies reprioritized activities, revised targets through discussions without having any implication on resources.
Regular check-ins also helped us finalize the cadence and data collection mechanism for different indicator types including complex ones. One of the indicators that the grantees were concerned about collecting data was the income generated for the beneficiaries from the program. Through joint discussions, we came up with a methodology to collect income data from a representative sample of beneficiaries to ensure data quality and reduce the burden on grantees.
4. Data Triangulation Mechanisms Help
Setting up data triangulation mechanisms, especially for complex indicators, helps in improving data quality. For example, income data both at the women beneficiary level and the collective level are helping us to catch inconsistencies and validate data.
5. Regular Interaction Helps Grantees’ Cross Learning
Regular discussions help in capturing the best practices of one grantee and exploring its feasibility with other grantees. For example, one of our grantees is implementing an ERP system for their organization and the peer learning platform helped the grantee to share their experience with others who wish to strengthen their compliance systems.
6. Monitoring Systems Help Build Evidence Portfolio
As grantees improve their data systems, they also generate an evidence portfolio or track record to showcase their impact to their stakeholders. We are conscious that the formative and impact evaluation and learning that draw from the monitoring systems should also help grantee project implementers.
Cover Image: © WASSAN
This article was first published on 3ie’s Evidence Matters blog. Read the original piece here.
About the Author
Morchan Karthick is a Senior Data Scientist with LEAD. He is an evaluation and data analytics expert with more than eight years of research and consulting experience in Asia and Africa. Karthick has conducted multi-country research and evaluation programs to assess various government schemes. His core competencies are data analytics solutions, impact evaluations, M&E and strategic consulting.