“Living on a remote island in Pulicat, it is difficult for us to take people to a hospital during medical emergencies. Just last month, a village resident suffered a snake bite but passed away while he was being transferring to a medical facility”
Mani* — a 34-year-old, Irukum Village, Pulicat
34-year-old Mani* lives in Irukum village in the Pulicat Lake region and catches fish for a living. He informs us that the nearest hospital is several hours away from their island, and this is a considerable challenge during medical emergencies. When we probe further on the time taken to reach the hospital, Mani’s estimate is “more than 45 minutes”. On being asked how far the hospital is located, he answers “It should be around 10 -15 kilometres”. Through our scoping activity in the same village, we find that Mani overestimated both the time and distance to reach the nearest hospital.
During our fieldwork, we have come across several such instances. While some respondents can accurately estimate distances and time taken to reach the nearest infrastructure facilities like hospitals, bus stands and railway stations, we find that a majority of respondents are prone to underestimation or overestimation. This trend varies across different socio-demographic groups. These issues with self-reported data lead to biases and measurement errors, resulting in inaccurate information for policymakers to plan optimal interventions for infrastructure development.
Self-reported data on time and distance to reach different nearest infrastructure facilities produce underestimated, overestimated and rounded-off data points. In order to address the challenges of misreporting and providing high-quality data on time and distance estimates, we propose an alternative to traditional data collection through Google API services [1]. As part of a study of enterprises conducted by LEAD, researchers used Google API to capture the data on distance to the nearest facilities from respondents’ enterprise geolocations. Primary data was collected from 1,238 enterprise owners in the districts of Bangalore and Mandya in Karnataka and Dhanbad and Garhwa in Jharkhand. We further compared Google API data on time and distance taken to reach the nearest infrastructure facilities with self-reported data captured through surveys.
Key Findings:
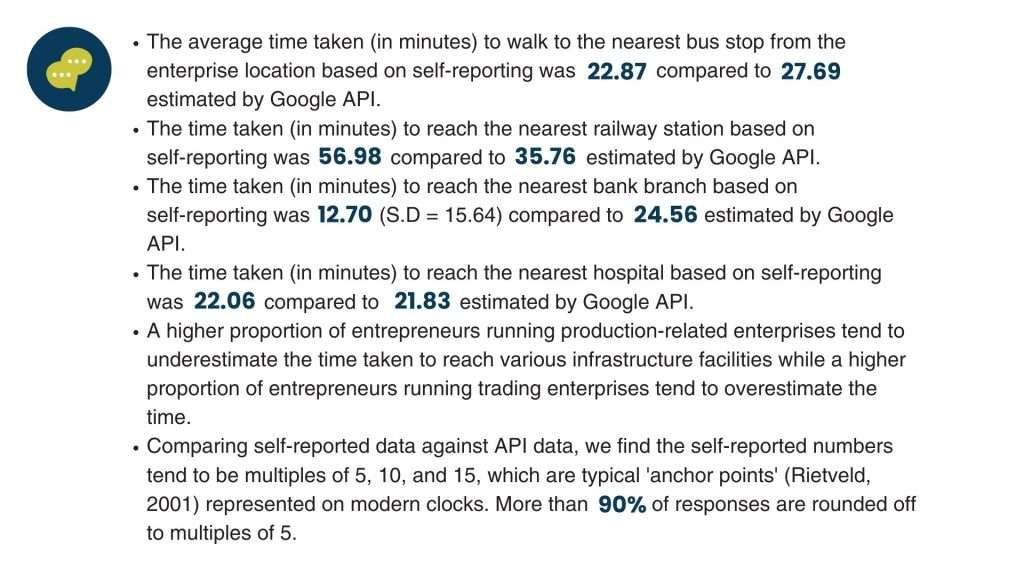
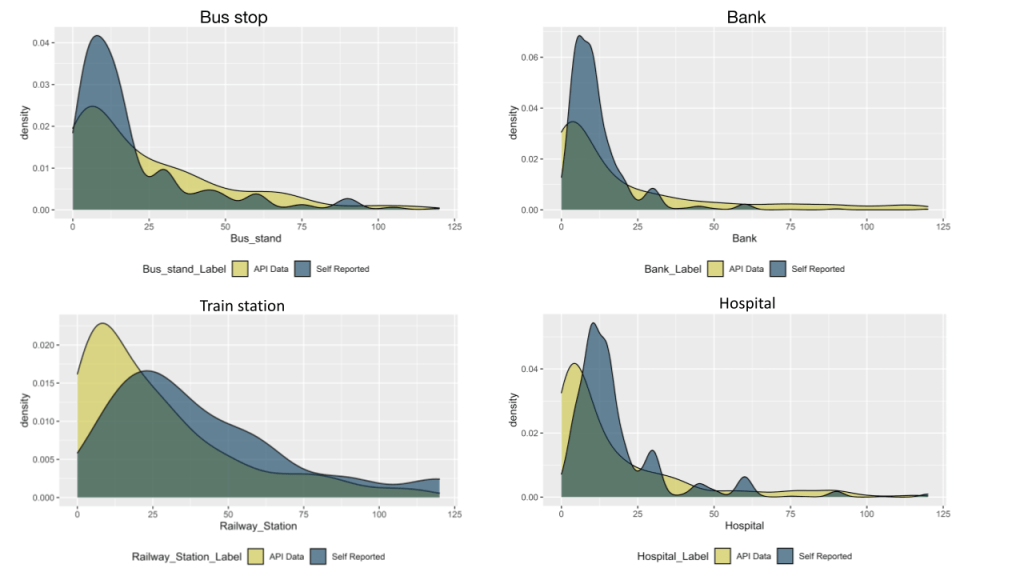
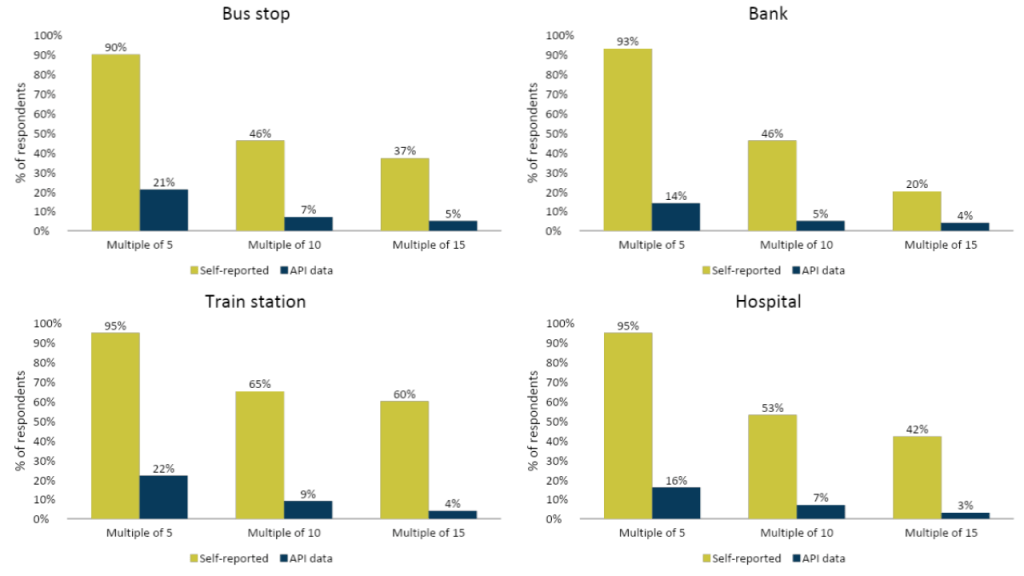
What are the implications of this approach for social surveys and potential use cases?
Solely depending on primary surveys for capturing an array of information from respondents using lengthy and time-consuming questionnaires burdens respondents. Such an approach may pose challenges such as non-response, attrition and misreporting of information(over/underestimation). Through our research, we present an innovative solution to improve the accuracy of data, which may be difficult to capture through traditional primary surveys and improve the overall quality of survey data.
Capturing accurate demand-side geospatial data on access to infrastructure has implications for reducing spatial inequalities in healthcare, transportation, financial services and other key determinants of economic development. Optimal planning in locating health facilities has improved healthcare utilisation and patient care [2]. Similarly, enhancing mobility through public transport creates education and employment opportunities. As social science and field research evolve, innovative data techniques that provide more accurate estimates of distance and time can be leveraged to improve last-mile access to essential services.
This blog is based on an article published in the Asia Pacific Journal of Evaluation, Vol. 01, Issue 01. Read the full article here.
Endnotes
[1] https://cloud.google.com/apis/docs/overview
[2] https://bmchealthservres.biomedcentral.com/articles/10.1186/s12913-021-06226-5
*The respondent’s name has been changed to protect his privacy.
About the Authors
Nelson Mathews is a Senior Research Associate with the Small, Growing Businesses and Employment vertical at LEAD. His primary research interests are social protection, public service delivery, and program evaluation.
Shruti Kakade is a Senior Data Associate with the Small, Growing Businesses and Employment vertical at LEAD. She is passionate about data-driven decision making, and currently volunteers for the UNDP data futures team and the Women in Econ/Policy group.