In the fast-changing world of social impact research, the emergence of Artificial Intelligence (AI) — especially Generative AI — presents exciting new possibilities, especially for enhancing the efficiency of workflows in qualitative research. This technological breakthrough grants researchers access to tools that can improve the efficiency of processes and unlock insights from complex, unstructured data like never before. In this article, we talk about how we are tapping into the transformative potential of AI in social research. We will explore its applications and benefits in qualitative research, along with the ethical considerations it raises.
The Traditional Workflow
The traditional journey of qualitative research starts with data collection, where insights are gathered through human interactions and literature review. These interactions are documented and organized digitally for a comprehensive analysis. Coding reveals themes and patterns, leading to the interpretation that connects findings to observed behaviours and trends. The iterative process refines and adapts the research scope based on new insights, highlighting the depth and flexibility of qualitative research.
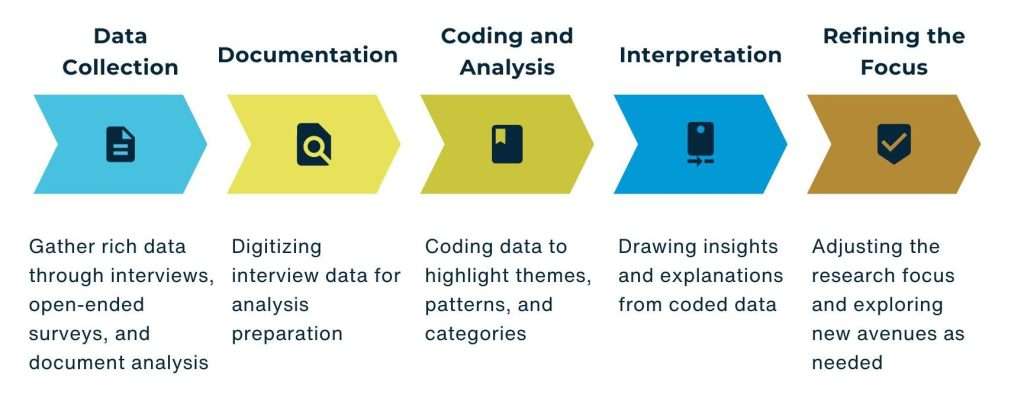
AI Strategies to Enhance Qualitative Research Workflow
AI solutions can be embedded into qualitative research workflows in three critical areas:
- Documentation: Transcribing qualitative interviews traditionally requires significant labour and time, with variations in output quality, which largely hinge on the transcriber’s skill. For instance, OpenAI’s Whisper, an open-source neural network, stands out for its robustness and accuracy in English speech recognition. This Automatic Speech Recognition (ASR) system is trained on 680,000 hours of diverse, web-sourced data, showing resilience to accents, background noises, and technical jargon. It supports transcription across multiple languages, and offers translation to English, significantly enhancing the efficiency of multilingual research projects. Without speaker diarization, the output merges into a single paragraph, obscuring who said what and often causing confusion. This typically necessitates post-processing, usually done manually. However, GPTs—or GPT bots—present a viable alternative. These customizable bots utilize a knowledge base that can be tailored with specific formatting examples. Through intelligent prompt engineering, GPTs can reliably replicate results consistent with these examples. While the standard ChatGPT platform can achieve similar outcomes, GPTs generally deliver more precise and consistent outputs for such tasks.
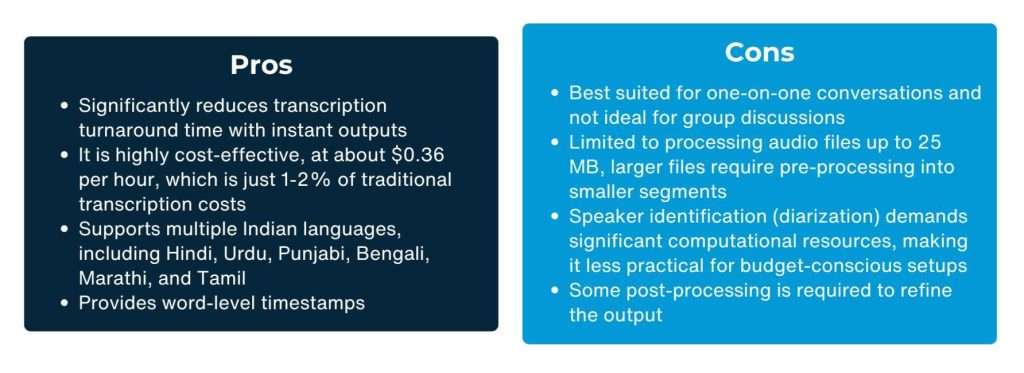
- Coding and Analysis: Traditionally, researchers using qualitative analysis software meticulously review the entire draft, categorizing relevant evidence into themes or nodes through open coding. An alternative method involves querying: researchers input a set of keywords or phrases linked to a specific theme, including all variations of the keyword. The software then highlights all sections where the keyword appears for further review and categorization. AI can enhance this process by removing the need for coding expertise, allowing queries to be conducted in natural language and simplifying the workflow. While this approach requires some adjustments to the documentation process, it remains a promising alternative. Atlas Ti, a leading platform in qualitative analysis, is also exploring this capability, though it remains a work in progress. The growing integration of AI in qualitative analysis appears inevitable.
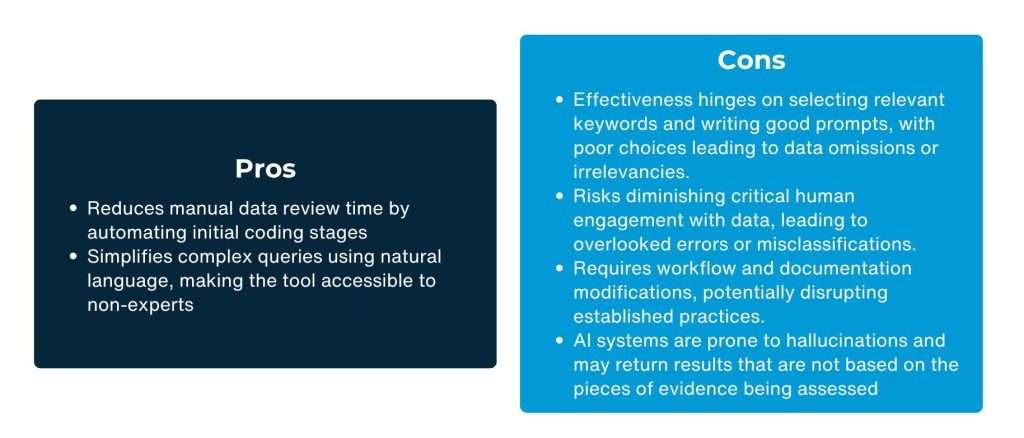
While exposure and practice can mitigate some challenges, AI hallucinations remain a concern. This issue can be addressed to a certain extent by setting the temperature attribute closer to zero. At lower temperatures, the model’s responses are more deterministic and conservative, ensuring more factual answers. Additionally, Retrieval Augmented Generation (RAG) systems can enhance verification by returning specific parts of documents from which evidence is drawn, providing an extra layer of validation for the results.
- Interpretation: Human intervention remains crucial for interpreting data, yet AI can uncover latent insights and patterns within qualitative evidence. AI simplifies two primary types of analysis: assessing the co-occurrence of insights or traits, and streamlining sentiment analysis. These tasks traditionally demand technical expertise and coding skills, but AI can reduce them to simple prompts that anyone can execute. Effective prompt crafting, which hinges on clear and straightforward instructions, is essential for accurate results. But since this is done in natural language it is easier to adapt for an average researcher compared to mastering complex algorithmic analysis. AI tools like ChatGPT or Google’s Notebooklm further simplify the process, enabling the analysis of multiple documents simultaneously. Using enterprise subscriptions or APIs is recommended, as they ensure that data access is restricted to the user, thus enhancing data privacy. Additionally, it is advisable to remove personally identifiable information from the data before uploading it for analysis on AI platforms.
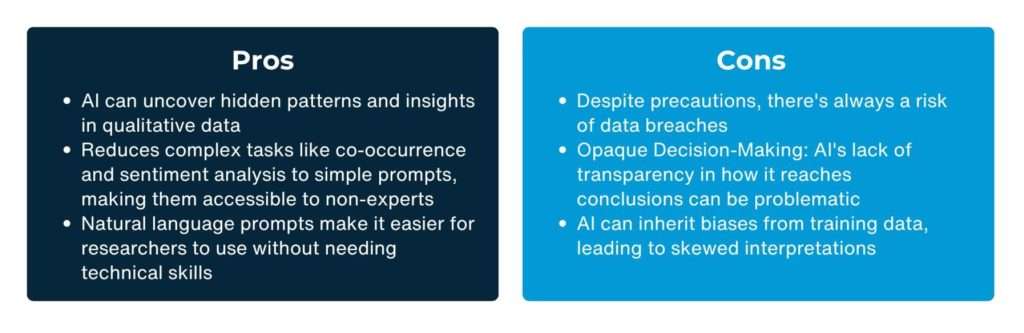
In essence, the integration of Artificial Intelligence in qualitative research is not just a technological upgrade but a paradigm shift that enhances the depth, speed, and accuracy of qualitative research. By effectively harnessing AI tools such as Whisper for transcription and GPTs for data processing and analysis, we can set new benchmarks in how qualitative research is conducted and insights are generated. Nevertheless, the importance of balancing these innovations with ethical considerations and human oversight remains paramount. These integrations must be pursued with caution and responsibility, ensuring that the applications augment human expertise rather than replacing the critical human touch that defines and enriches qualitative research.
About the Authors
Gaurav Kumar Sinha is a Senior Manager with the Data Innovations vertical at LEAD. Gaurav has over eight years of experience in process and outcome measurement across diverse themes like financial inclusion, WASH, education, public health and clean energy. An avid traveller, Gaurav unwinds by cooking and exploring virtual reality.
Aditya Tomar is a Data Manager with the Data Innovations vertical at LEAD. He is currently pursuing an integrated Master’s Degree from Sardar Vallabhbhai National Institute of Technology, Surat and holds a Bachelor’s Degree in Programming and Data Science from the Indian Institute of Technology, Madras. When Aditya clocks off, he enjoys staying updated on global innovations and finds solace in spiritual literature.